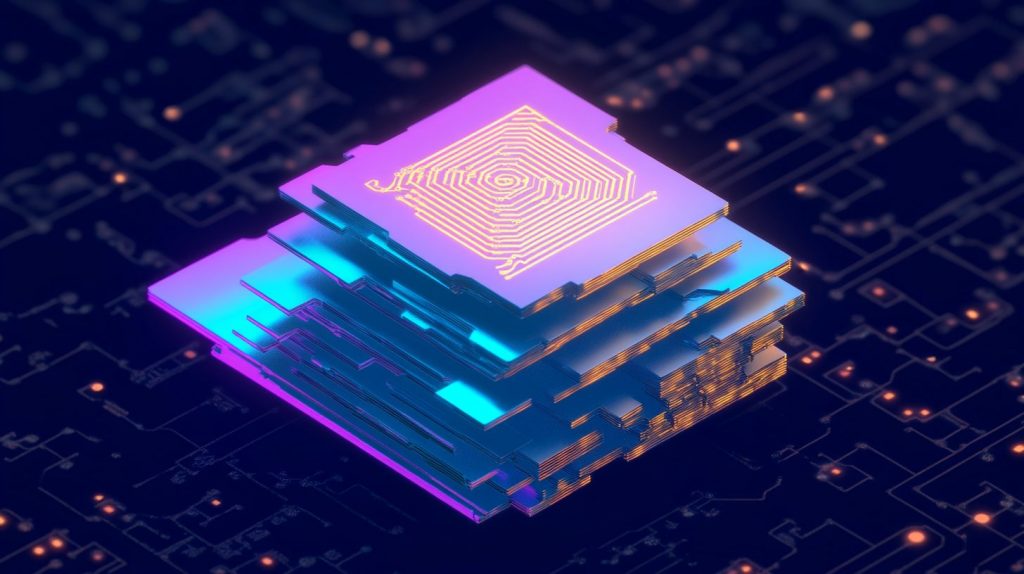
Adaptive Computing Systems as Accelerators of the AI Revolution
About Prof. Andreas Koch, PhD
Prof. Andreas Koch is head of the Embedded Systems & Applications Group (ESA) at TU Darmstadt and a founding member of hessian.AI. His academic career led him from a diploma in computer science at the TU Braunschweig via a postdoctoral position at UC Berkeley back to Germany, where he habilitated at the TU Darmstadt. There he founded his own department and established himself as a leading expert in adaptive computing systems.
Koch’s Focus: Adaptive Computing Systems
Koch’s research covers a broad spectrum: from tiny embedded systems that are, for example, attached to bridges to programs for the world’s fastest supercomputers, like those used in the search for the COVID-19 drug. His main focus is on the development of hardware that is specifically tailored to problems that cannot be solved efficiently with standard hardware.
Koch concentrates on adaptive computing systems in which parts of the hardware can be specially adapted to the requirements of the software. This approach opens up new possibilities, particularly in the field of machine learning, and offers solutions to challenges in terms of energy efficiency and speed.
As an example, Koch mentions a project for the automotive industry funded by the Federal Ministry of Education and Research (abbreviated: BMBF). The project involved the optimization of machine learning techniques in audio signal processing with the help of a specially adapted RISC-V processor. The adaptations led to a more than two-fold increase in performance and a significant reduction in energy consumption of 30 percent.
“Hardware is hard”
In Koch’s experience, hardware design is a particularly challenging task. He quotes his now deceased colleague Professor Wolfgang Strasser from Tübingen: “Hardware is hard”. In contrast to software development, where errors can often be rectified quickly, the smallest deviations in hardware design can lead to serious problems.
Koch emphasizes that the tools for chip design are among the most complex software solutions of all. This complexity makes working with them particularly challenging and explains why hardware design is such a specialized field.
AI design for AI chips
One new approach Koch highlights is the use of machine learning in chip design. In our conversation, he refers to a Google keynote he attended that highlighted the impressive opportunities that arise when machine learning is applied to chip design. In particular, the arrangement of elements on the chip is optimized by AI, which leads to more efficient and cost-effective designs, the researcher tells me.
Koch sees huge potential in AI assisted chip design, but points out that this technology is best suited to companies that already have extensive collections of chip designs. For smaller companies or start-ups, this could pose a more challenging task.
Koch sees Great Potential for more Efficient Hardware
Koch’s research enables the use of AI models – including completely new architectures – beyond the limits of conventional hardware. This could enable large and complex problems to be solved with significantly reduced energy consumption. This is particularly relevant at a time when energy efficiency and sustainability are becoming increasingly important.
Koch and his team are also investigating how AI-specific hardware can be used efficiently for traditional scientific computing, such as flow simulations. This synergy between machine learning and scientific computing could have far-reaching implications.
hessian.AI has proven to be an indispensable resource for Koch’s research. Access to powerful hardware via the Hessian Supercomputer 42 enables him to tackle innovative projects that would not be possible without these resources, says Koch.