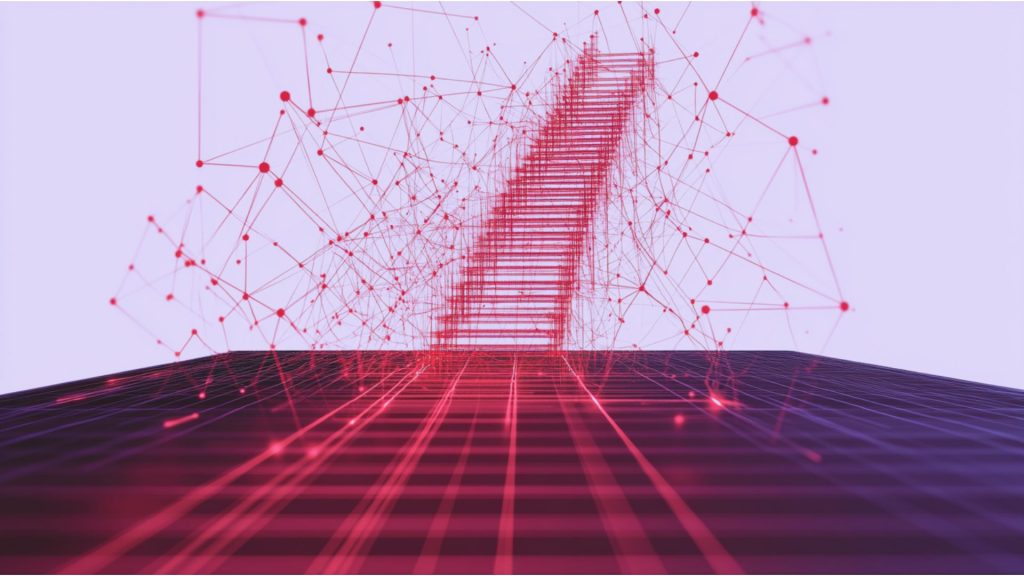
Climbing the Ladder of Causality: AI’s Journey Beyond Mere Correlations
About Florian Busch
Florian Busch is a PhD student at the Technical University of Darmstadt and hessian.AI. After completing his bachelor’s and master’s degree in computer science with a focus on artificial intelligence and machine learning, he is now researching how artificial intelligence can understand cause and effect.
The ladder of causality and the limits of AI
In our interview, Florian Busch emphasizes a threefold division in causality research that goes back to the work of the well-known researcher Judea Pearl.
In his “Ladder of Causality”, Pearl distinguishes between:
- Associations: These involve predictions based on passive observations and therefore only constitute correlations.
- Interventions: These involve a change to what already exists, i.e. a prediction of what will happen if you specifically intervene in a system.
- Counterfactual statements: These deal with the question of what would have happened if certain conditions had been different, i.e. with what could have been.
Busch is researching methods that will enable AI to understand which causes lead to which effects – in other words, to understand levels 2 and 3 of the causality ladder. This is in contrast to most current AI models, which only map correlations and are therefore at level 1.
Sum-Product Networks: A tool for researching causality
A key instrument in Busch’s work is the so-called Sum Product Networks (SPN). These models are used to calculate probabilities and are originally only geared towards correlations. Busch’s aim is to adapt these networks so that they can also process interventions and counterfactual statements in order to provide a comprehensive picture of causality in AI systems.
Busch sees a major challenge for his research in the fact that causal models largely rely on very specific assumptions that are often not fulfilled in practice. The methods work well for simpler problems with few variables, but often no longer work for more complex problems with many variables.
His aim is therefore also to improve the scalability of the models so that they can be used for more complex problems with many variables, as is often the case in the real world.
In our conversation, Busch also emphasizes the positive role of hessian.AI for his research, especially with regard to the freedom in research work and the networking opportunities. The organization enables him to engage in productive collaborations and offers platforms such as AI-Con that provide insights into various fields of research.
Causality in medicine and climate research
Busch’s research has the potential to make an important contribution to the development of AI. He points out that many areas of AI currently manage without causality, but that a deeper understanding of causal relationships is essential for more intelligent and more widely applicable AI systems.
He sees an interesting field of application in medicine, for example, where such models could make the effect of medication comprehensible and distinguish it from other influences such as the placebo effect. The models could also be helpful in the fight against climate change. “There is a lot of data and knowledge, but if you had a model that could learn causal relationships, both interventions and counterfactuals, then you could ask new questions and understand cause and effect,” says Busch.