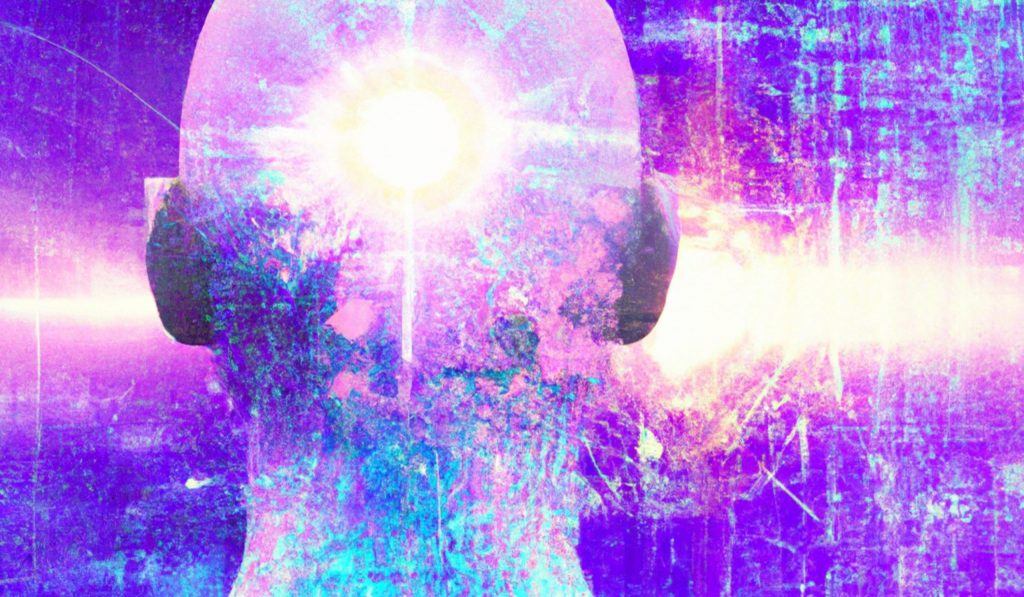
Better interaction with artificial intelligence
Darmstadt, March 10, 2023. A team of researchers from TU Darmstadt, hessian.AI and the German Research Center for Artificial Intelligence has presented a method that greatly simplifies giving human feedback to learning software. The work appears in the current issue of Nature Machine Intelligence.
The whole world is amazed at ChatGPT: the speech AI answers questions, writes papers and even spits out poems. Its human writing style contributes to the success of the adaptive software. For Felix Friedrich from the Technical University of Darmstadt, ChatGPT is an example of how important interaction between humans and machines is. This is because the software’s eloquence comes not only from the millions of digital texts with which it has trained itself, but also from refining this training in dialogue with humans.
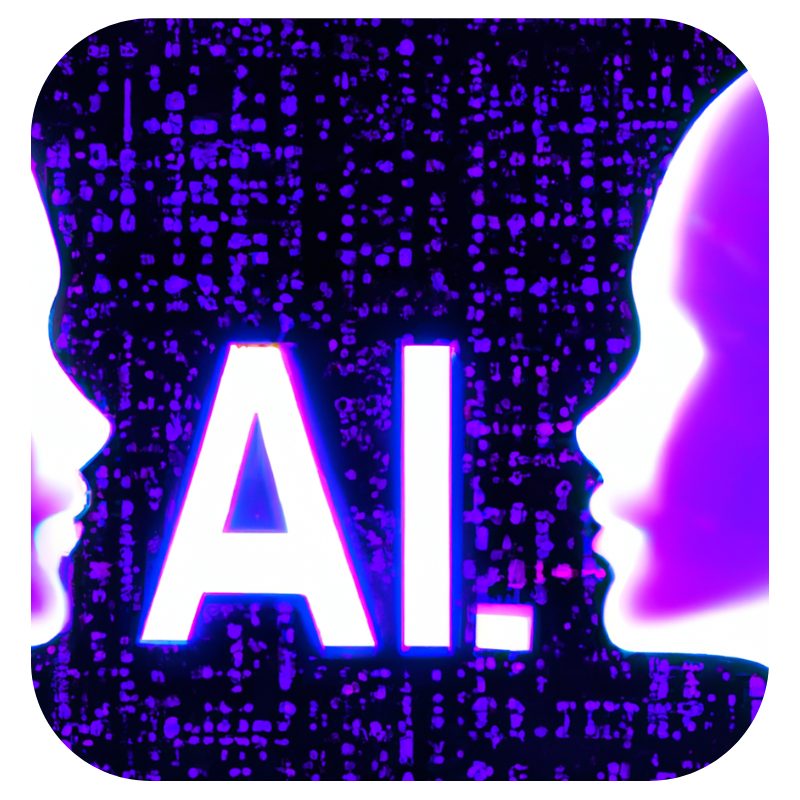
Many AI algorithms already benefit from feedback from humans, as Darmstadt researchers led by Prof. Kristian Kersting from the Department of Computer Science have shown in recent years. However, Felix Friedrich, who is doing his doctorate under Kersting, is convinced that the potential is far from exhausted. Now, together with colleagues, the computer scientist is presenting a method that considerably simplifies the giving of human feedback. The work appears in the current issue of the renowned specialist journal “Nature Machine Intelligence”.
To communicate with machines, you have to understand them. But this is difficult with the currently most used form of AI, so-called deep learning. Deep learning is inspired by neural connections in biological brains. Large deep-learning networks have billions of connections between virtual neurons. It is difficult to understand which signals contribute to an AI decision and which do not, i.e. how the software arrives at its result. It resembles a black box.
To communicate with machines, you have to understand them. But this is difficult with the currently most used form of AI, so-called deep learning. Deep learning is inspired by neural connections in biological brains. Large deep-learning networks have billions of connections between virtual neurons. It is difficult to understand which signals contribute to an AI decision and which do not, i.e. how the software arrives at its result. It resembles a black box.
“Often people don’t ask about it as long as the AI works,” says Friedrich. But this makes it easy to overlook when the AI takes so-called “shortcuts” that can lead to errors. What are such shortcuts? Deep learning is often used to recognise certain objects in pictures, for example: Polar bears. For training, you put a lot of pictures of polar bears in front of it, which is supposed to teach it what makes a polar bear based on the similarities. Now it may be that the AI makes it easy for itself. If there was always snow in the background of the training pictures, then it takes this snow in the background as the characteristic of a polar bear picture instead of the polar bear itself. If a brown bear appears in front of a snowy landscape, the AI mistakenly recognises it as a polar bear. With the help of a method called Explainable AI, such errors can be detected. The deep-learning algorithm shows which patterns it used for its decision. If they were the wrong ones, a human can report this back to the AI, for example by showing the correct patterns (for example the outline of the bear) or by marking the wrong ones as wrong. The researchers call this feedback process Explanatory Interactive Learning (XIL).
The Darmstadt researchers have now examined several existing XIL processes. “No one had ever done this systematically before,” says Felix Friedrich. The researchers meticulously analysed how the interaction with the computer proceeds in the respective methods and where efficiency gains are possible. They distinguished which components of an explanation are important and which are not. “It turned out that it is not necessary to report thousands of markers back to the machine, but that often a handful of interactions are enough,” says Friedrich. It is often enough to tell the AI what does not belong to the object instead of defining what does. Specifically, the researchers gave an AI the task of recognising a handwritten “1”. However, in the corner of each training image was also a small square. If the algorithm mistakenly took this as a relevant feature for the “1”, it was enough to mark the square as not belonging to the object. “This is much more effective, because it is generally difficult to define what distinguishes a kingfisher, for example,” Friedrich explains.
The Darmstadt researchers have distilled a strategy (“typology”) from the XIL processes they studied, which can be used to efficiently remedy the shortcut behaviour described. Felix Friedrich and his colleagues are already working on the next project, which aims to improve the interaction between humans and machines. This time it’s about text-to-picture generators, which often have a “bias”. When instructed to display a “firefighter” (the gender-neutral English word for “fireman”), they usually generate images of white men wearing firefighter helmets, which is because images of male, white firefighters dominate the training data. Through human interaction, the Darmstadt researchers want to make the training data for such image generators more diverse, by filtering, but also by adding images, for example of coloured firefighters. Felix Friedrich is thus coming closer to his goal of “human-guided machine ethics”.
The authors of the publication conduct research at the Technical University of Darmstadt, at the Hessian Centre for Artificial Intelligence, at the German Research Centre for Artificial Intelligence (DFKI). The research is part of the cluster project “3AI – The Third Wave of Artificial Intelligence”.