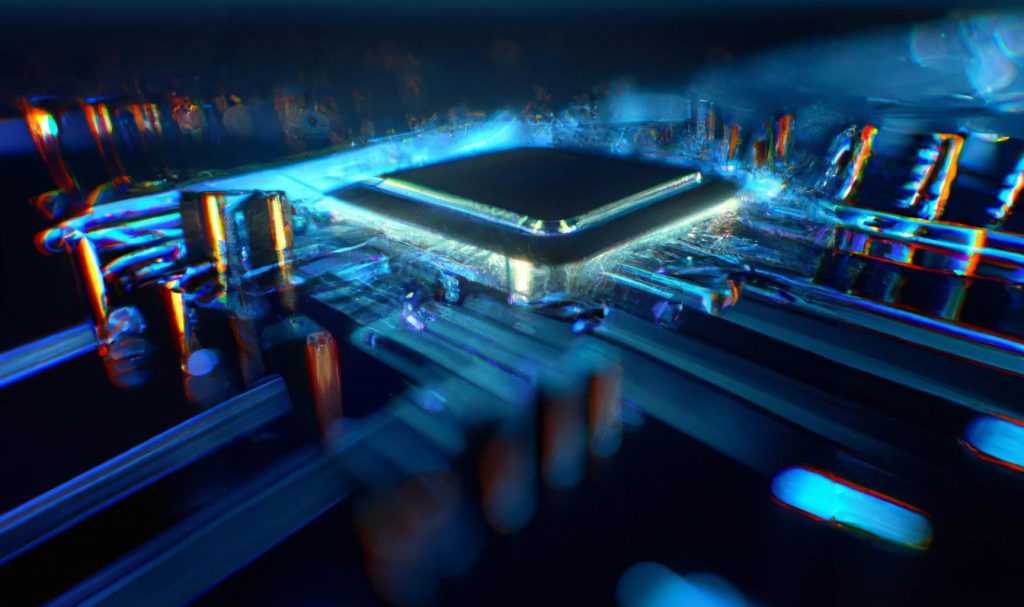
Breaking the limits of AI hardware
About Prof. Dr. Li Zhang
Prof. Dr. Li Zhang is a researcher at the Department of Electrical Engineering and Information Technology at the Technische Universität Darmstadt. She received her Ph.D. from TU Munich, where she was a group leader for Heterogeneous Computing from 2018 to 2022.
Since 2022 she is Assistant Professor on Hardware for AI at TU Darmstadt.
Understanding why AI works
Artificial intelligence has become an integral part of our daily lives, from recommending products we might like to use, to detecting anomalies in medical scans, or generating an image from words. But despite the prevalence of AI, there is still much to understand about it.
Particularly with complex and multi-layered models, it can be difficult to understand exactly how an AI system arrives at its final predictions or decisions. Neural networks are even considered “black boxes” because their decision-making process remains partly nontransparent.
Zhang’s research focuses on understanding the nature of artificial intelligence from a hardware perspective: her hardware and algorithmic designs enable more efficient and advanced models that provide increased interpretability for AI.
“My goal is to explore the relationship between logic design and deep neural networks,” Zhang says, adding that her research focuses on the hardware perspective. Analogue and digital circuit designs play a crucial role in the implementation of AI systems by providing the computational resources needed to run and interpret AI algorithms and models, such as neural networks – and both have their strengths and weaknesses.
Her primary goal is to develop hardware and algorithmic designs that can utilize both circuits’ strengths while mitigating their limitations. Analog circuits, for example, are more energy-efficient and faster, but they lack the accuracy and robustness of digital circuits: “Analog accelerators can be up to 70% faster compared to digital ones, but they are less accurate and not reliable enough”, says Zhang.
However, compute demands are increasing faster than hardware can keep up with: “The development of AI is fast. But hardware for AI cannot catch up with that.”
Paving the way for sustainable AI systems
Increasing the efficiency and computational power of hardware and algorithmic designs would allow researchers to gain better insights into how AI reaches a conclusion, optimize their performance, and develop more advanced algorithms that take advantage of the hardware’s capabilities.
Currently, most AI systems utilize Graphics Processing Units (GPUs) to train neural networks. This approach has one key drawback, according to Zhang: “The high power consumption of GPUs is a very, very large issue”.
Her hardware and algorithmic designs offer researchers and industry a way to train models with better power efficiency, ultimately leading to more sustainable AI systems.
Collaboration is a key aspect of Zhang’s research, and she sees interdisciplinary research as essential to developing sustainable, efficient and robust AI systems. For Zhang, hessian.AI provides a means for researchers from different disciplines to collaborate, find synergies and gain a deeper understanding of AI.