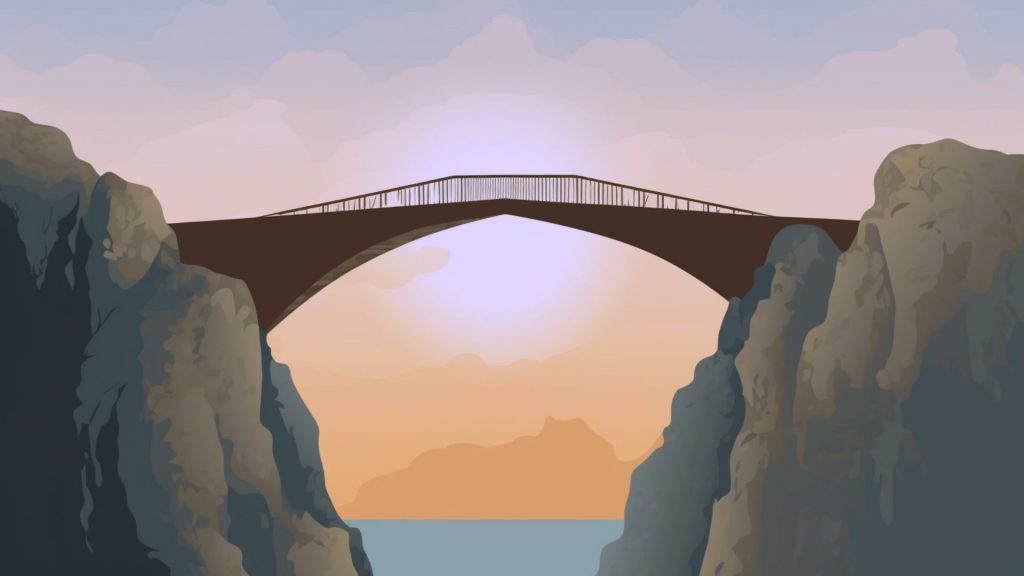
Bridging the Gap: Reinforcement Learning’s Real-World Solutions
About Carlo D’Eramo
Carlo D’Eramo began his academic career with a Master’s degree in Computer Engineering with a specialization in Artificial Intelligence in Milan. He received his PhD in Italy in the field of reinforcement learning, a subfield of machine learning that deals with how software agents should act in an environment to achieve specific goals.
After his doctorate, D’Eramo spent some time as a postdoc at the Technical University of Darmstadt, where he later headed the Hessian AI group LiteRL. Since November 2022, he has been Professor of Reinforcement Learning and Computational Decision-Making at the University of Würzburg and continues to work in Darmstadt to supervise his doctoral students.
Focus on reinforcement learning
D’Eramo’s research focuses on reinforcement learning, a method of machine learning in which an agent learns through trial and error and is rewarded to perform certain actions in a given environment. This method has a wide range of applications, from optimizing chess strategies to investing in the stock market.
One of the biggest challenges in this field is bridging the gap between theory and practice, according to D’Eramo. “We are good at solving a single problem, but if the problem changes or the data is noisy, the systems no longer work,” says the researcher. AI systems must therefore become more adaptable and robust, especially in situations where the environment or the task changes.
Multi-task reinforcement learning should make systems robust
A key aspect of D’Eramo’s research is therefore multi-task reinforcement learning. By learning different tasks, an AI model improves its ability to generalize and adapt to unforeseen problems. This adaptability is crucial for the development of an artificial intelligence that can survive in the real world, says the scientist.
However, working with deep learning methods is a major challenge, especially in reinforcement learning, as these methods often work with many parameters and extensive tests with so numerous variables can quickly take several days. D’Eramo is looking for more efficient models that require fewer resources and can be trained more quickly – so that new methods can be tested and further developed faster.
He sees his current dual role as professor at the University of Würzburg and head of the LiteRL group at TU Darmstadt as an interesting opportunity to establish research collaborations between the two universities. He sees hessian.AI as a good role model: the center is open to various topics and enables interesting and fruitful collaborations, for example between his field of research and lifelong learning.
“We should not forget the importance of theory “
With his work, D’Eramo aims to create AI systems that are more adaptable and therefore more generalizable. In the long term, he hopes to promote the development of a general artificial intelligence with his research.
When he is not training models in simulations, the scientist also cooperates with researchers from the field of robotics. There, his methods could for example help to make robots more reliable. Also beyond robotics, better RL algorithms could help people in many areas, for example by making better recommendations for the treatment of patients based on their medical history, which differs from person to person.
But “we must not forget the importance of theory, we have to make sure by using mathematical theory that what we apply is safe,” D’Eramo tells me at the end of the interview. Research must always find a balance between the theoretical treatment of problems and maintaining an application perspective.