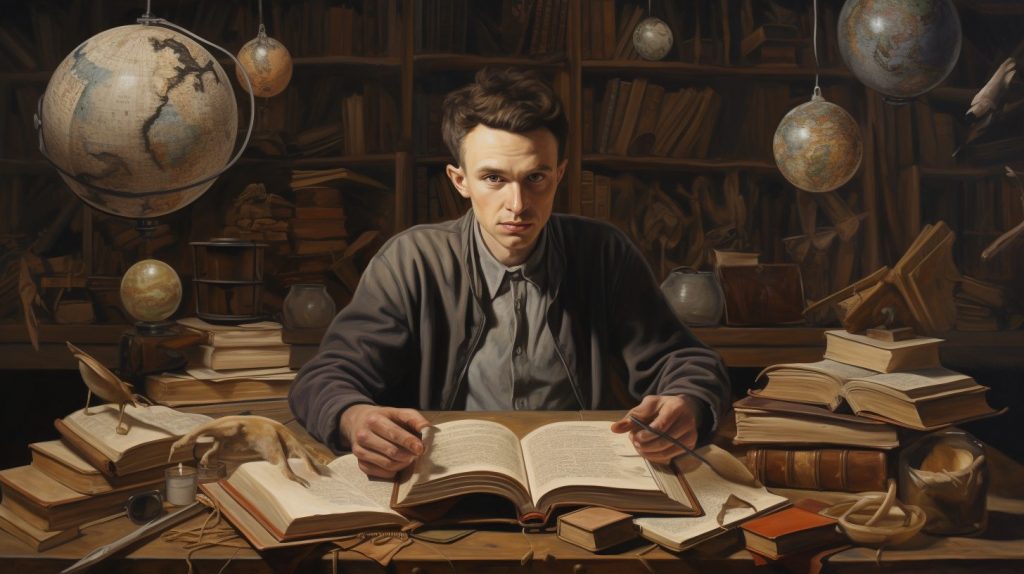
From static to lifelong learning in AI
About Dr. Martin Mundt
Dr Martin Mundt studied physics and worked on neuroinspired models in his master’s thesis. He received his doctorate in computer science from Goethe University Frankfurt in 2021.
He then moved to the AIML Lab at TU Darmstadt as a postdoc and has been Junior Research Group Leader of the Open World Lifelong Learning (OWLL) group at TU Darmstadt and hessian.AI since 2022.
People learn in a structured way – machines do not
Mundt and his OWLL group are working on the question of how AI systems can learn for life. Today’s AI methods train AI models with fixed data sets. However, the real world does not consist of such data that is always the same, says the researcher.
In the field of lifelong learning, Mundt is therefore looking for approaches that can continuously learn from the constantly changing data in a real application in such scenarios.
The problem: Modern machine learning methods are very unstructured and the systems learn individual elements of the data independently. Humans, on the other hand, learn in a structured way, says Mundt, for example first the easier concepts of a new language and then the harder ones that build on them.
Holistic approach to enable lifelong learning
However, this approach does not work easily with current methods. When an AI model learns a new concept, it usually forgets large parts of the first one – this is known in science as “catastrophic forgetting”.
Mundt is therefore looking for a holistic approach to lifelong learning that allows AI systems to learn in a structured way without forgetting, and furthermore to always decide whether new data really contain new concepts.
“That is the fundamental idea of my research: how can I learn continuously in a structured way and make robust decisions at the same time?” says Mundt. For this, he says, the current frontier of machine learning must be pushed and also combined with symbolic systems.
Transparency is central, says Mundt
Mundt sees the biggest challenge in the field of lifelong learning in finding a suitable approach that does not tackle the problem too broadly – i.e. does not require general intelligence right away – but also does not focus only on one problem, which is then no longer compatible with other systems.
That is why it is also important to ensure transparency in the research community, he says. The work in his field would have to clearly define which problems it solves and where it no longer works.
To support this process, Mundt has published a comprehensive overview of the last 30 years of AI research that highlights the different aspects of lifelong learning and proposes a transparency map on which researchers can show the different dimensions of their work and improve comparability.
Martin Mundt is also a board member of the non-profit organisation Continual AI, where he supports, among other things, the development of Avalanche, an end-to-end library for continuous learning.
hessian.AI as an enabler
hessian.AI is a central enabler for his research, says Mundt, unique in its organisation of researchers and incredibly helpful in the exchange with these experts from different AI fields. This exchange and the shared resources, such as access to data centres, are a basic prerequisite for his research.
It highlights the limitations of current AI systems, which require large amounts of data, energy and other resources, and could lead to approaches that move from static learning to lifelong learning, producing AI systems that are not focused on specific benchmarks but are directly relevant to the application.
AI systems that recognise whether they already know something could also help reduce current problems such as hallucinations in language models.
Finally, continuous learning systems can also enable a participatory process, says Mundt, more specifically the inclusion of different population groups in the learning and updating process of AI systems.