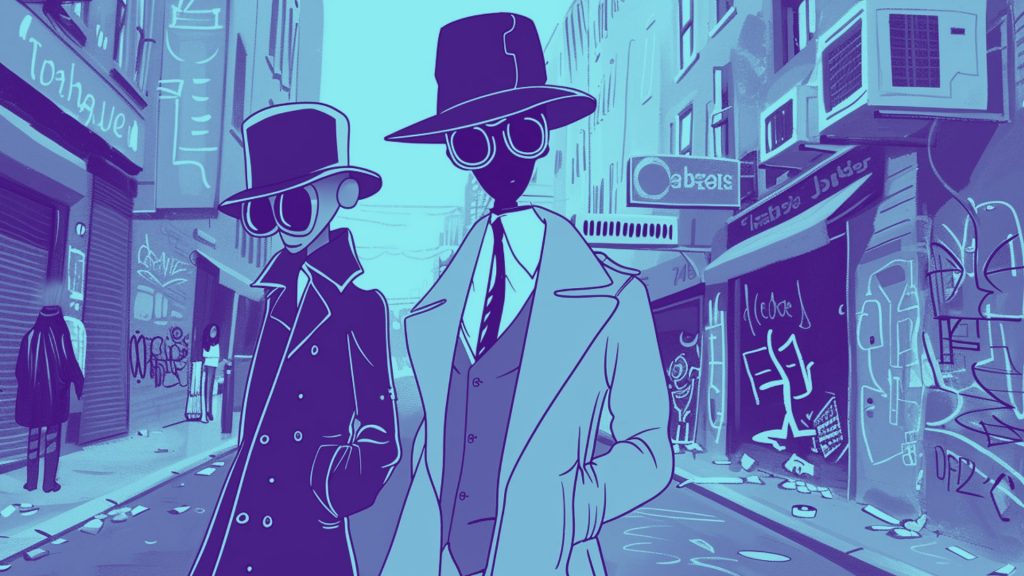
How adversarial reinforcement learning trains robust AI
About Aryaman Reddi
Aryaman Reddi is a PhD student working on multi-agent reinforcement learning and game theory. He began his academic career at the prestigious University of Cambridge, where he completed both his Bachelor’s and Master’s degrees in Information and Computer Engineering. During his studies, Reddi became interested in machine learning and mathematics. His Master’s thesis entitled “Deep Q-Learning for Congruent Non-Dominated Game Strategies” laid the foundation for his current research.
After his studies, Reddi gained practical experience at ARM, where he worked in the machine learning research department. His work focused on the optimization of neural networks for applications such as facial recognition on mobile devices.
Today, Reddi continues his research at the Technical University of Darmstadt, where he is a member of Professor Carlo D’Eramo’s LiteRL team. There, he is working intensively on the development of multi-agent systems that can solve complex problems more efficiently than previous technologies.
Focus on Adversarial Reinforcement Learning
One focus of his work is adversarial reinforcement learning. This involves training systems under difficult, hostile conditions in order to increase their robustness. One example project is robot locomotion, in which robots are trained to move against an opposing agent in simulated adverse environments such as ice or wind.
Another focus is cooperation in multi-agent systems. Here, Reddi is investigating how agents can work together more efficiently through improved communication. In the long term, this approach could lead to systems that can act effectively both as a team and in adverse scenarios.
In search of mathematical foundations
Reddi describes the gap between theory and practice as one of the biggest challenges in current AI research. Many of today’s machine learning methods are based on “fuzzy” ideas without a solid mathematical foundation, says Reddi – this leads to uncertainties in application and troubleshooting, as it can never be proven exactly where the problem lies.
“ChatGPT works well for creating simple texts, but often fails at more complex tasks like writing legal documents or analyzing long texts, such as about medical devices, and it’s hard to figure out why that is,” Reddi says. “There are so many moving parts that we only know work because of empirical evidence, but it’s hard to say they didn’t work because of this or that.”
His aim is therefore also to make a contribution to AI research that is simple and general enough to be implemented – and explained – by other researchers in multi-agent projects.
Multi-agent systems for communication networks
The hessian.AI research network plays a crucial role in Reddi’s work. By collaborating with other researchers from various fields such as computer vision and data management, he has expanded his knowledge and gained new perspectives for his research, Reddi explains in our interview.
When asked, he also talks about the potential social benefits of his research: the use of multi-agent systems in areas such as traffic flow and communication networks in particular could lead to more efficient and environmentally friendly solutions.
He also hopes that his research findings will help other scientists to develop more efficient algorithms and thus have a greater impact on the field of artificial intelligence in general.