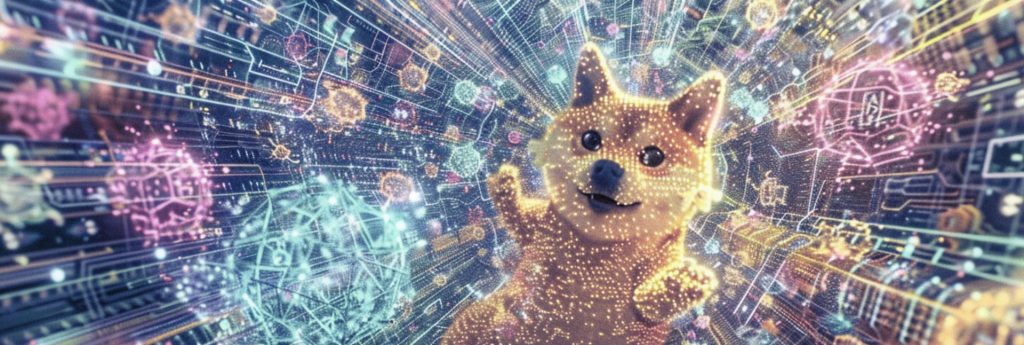
How AI should capture the complexity of memes
About Luke Bates
Luke Bates is a PhD student at the Ubiquitous Knowledge Processing Lab (UKP) and has a broad academic background, including two bachelor’s degrees in Linguistics and Japanese from Michigan State University and a master’s degree in Computational Linguistics from Seoul National University in South Korea.
His professional background includes a period as a cultural ambassador and English teacher in South Korea, as well as a six-month stint as a computational linguist in industry. Next, he then spent four years strengthening his research skills in a quantitative biology lab in Seoul, where he applied machine learning and computer vision algorithms to image and cell data.
Bate’s research focuses on the application of machine learning and natural language processing to combat the spread of fake news and conspiracy theories using multimodal methods. Moreover, he is also working on the application of artificial intelligence to support telemedicine.
Other research interests include the efficient use of transformer architectures and, in particular, the analysis of memes in social media.
Memes: more than just pictures with text
In our conversation, Bates emphasizes that memes are far more than just pictures with text: “Every single meme paper reduces memes to a picture and a sentence. Memes are more complex. He emphasizes that memes contain cultural and contextual references that go far beyond what traditional data sets capture.
In fact, memes can also work without text, as the example of “Leroy Jenkins” shows, says Bates.
In order to do justice to this complexity, Bates, and his colleagues, have used the website “Know Your Meme” as a resource for meme research. This website provides a comprehensive context to memes that was often lacking in previous studies. “We created this resource so that researchers can use AI models with this context,” says Bates.
Challenges of meme research
The biggest challenge in Bate’s research lies in the abstract nature of memes. They are constantly evolving and often contain intertextual references that require a deep cultural understanding. “Memes are often used for jokes. If you look at Indian culture but don’t know what Indian knowledge is, it’s impossible to understand,” explains Bates.
Bates’ research aims to develop practical and socially useful systems. One area he focuses on is the detection of conspiratorial content. He advocates systems that can warn against such content and thus contribute to the safety and well-being of society, for example by better recognizing and moderating dangerous content on social media.
hessian.AI , leading experts and “black magic”
In the interview, he emphasizes the importance of the Hessian AI Research Alliance for his work, especially the collaboration with leading experts such as Prof. Dr. Iryna Gurevych and Prof. Dr. Kristian Kersting, as well as the access to a powerful computing infrastructure.
Against the background of this available computational infrastructure, the researcher also reflects on current trends and challenges in AI research. He criticizes the current paradigm of large language models as “black magic”. Today’s large language models are difficult to control and understand and the most powerful methods are too extensive, not open source or often simply too expensive to be used transparently by researchers. This makes the research and development of e.g. models that understand when they don’t know something difficult.
Bate’s work in AI research, particularly in the context of memes, is an example of how complex, culturally and contextually rich data sources present new challenges and opportunities for AI.