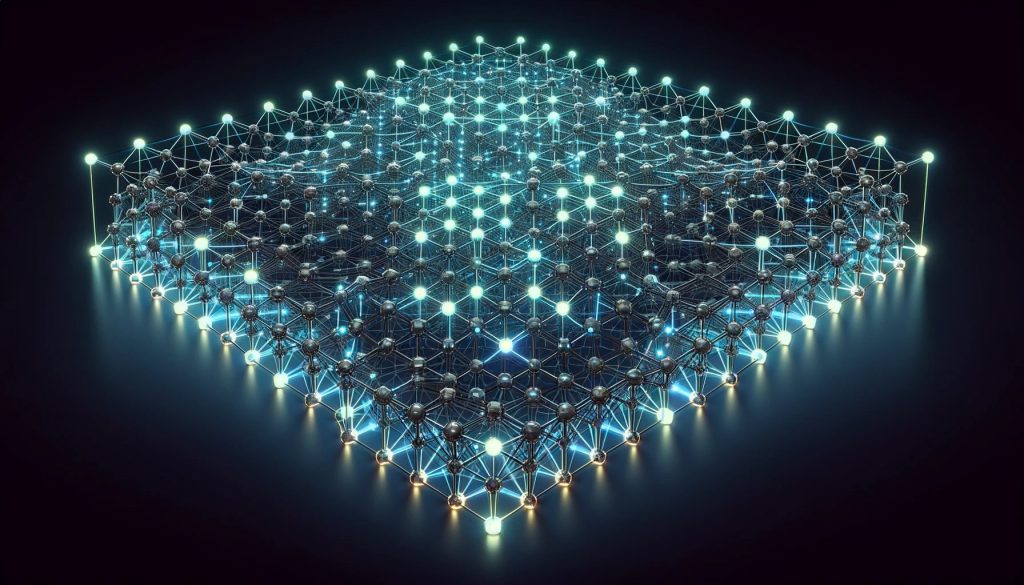
How Artificial Intelligence Learns to Understand Pandemics and Financial Systems through Mathematical Methods
About Christian Fabian
Christian Fabian is a Ph.D. candidate at the Self-Organizing Systems Lab at the Technical University of Darmstadt and a Research Assistant at hessian.AI.
In 2020, he completed his master’s degree in economics, followed by a master’s degree in mathematics in 2021. Since October 2021, he has been with hessian.AI in Darmstadt, conducting research at the Self-Organizing Systems Lab under the supervision of Prof. Dr. Heinz Koeppl since March 2022.
Reinforcement Learning for large networks of actors
In his research, Fabian focuses on “Reinforcement Learning for Mean Field Games.” The goal is to predict the collective behavior of very large groups of individuals using machine learning methods. This often involves hundreds of thousands or millions of actors.
Examples of such scenarios include the spread of a pandemic in the population, financial markets, biological swarm systems, and social networks. Models need to be developed that work for such large numbers of participants.
Standard reinforcement learning procedures reach their limits in such multi-agent scenarios. Therefore, Fabian specializes in the use of so-called Mean-Field Approximations, which are intended to provide a simplified understanding of complex interactions.
Graphons help understand complex networks
Specifically, Fabian uses reinforcement learning in conjunction with so-called graphons. A graphon is a tool used in mathematics to examine and understand large, dense networks, such as social networks.
Instead of considering individual connections, such as who is friends with whom, a graphon provides a simplified overview. This allows Fabian to model how individuals are influenced by their network neighbors and how they spread information, even with a high number of participants.
In the case of a pandemic, for example, the method could map personal contacts through which infections spread. The model can then learn what protective measures individuals take depending on the infection status of their neighbors and make predictions for further development.
A current challenge Fabian is working on is to make the graphon models more realistic. The structures used so far do not closely resemble real social networks enough to enable precise predictions.
In his publications, he has already taken steps in this direction. The next goal is to develop learning methods that can depict real structures of social networks. Another goal is to combine current models with Inverse Reinforcement Learning, for example, to explain the behavior of large groups of people in cognitive science.
Applications in finance, public health, and robotics
hessian.AI provides a conducive environment for this research direction. Regular exchanges, conferences, and shared resources such as computing power enable the transfer of ideas between different areas of AI.
His area benefits from encountering real problems while delivering scalable algorithms that others can build on—a collaborative process that hessian.AI aims to facilitate deliberately.
Potential application areas include, in addition to estimating the impact of public health measures on the dynamics of pandemics, predicting market behavior and systemic risks in the financial world, or controlling drone swarms.
Such models could help politics and economics make informed decisions in the future—such as containing pandemics or regulating financial markets. AI systems that accurately model collective behavior have the potential to provide significant societal benefits.”