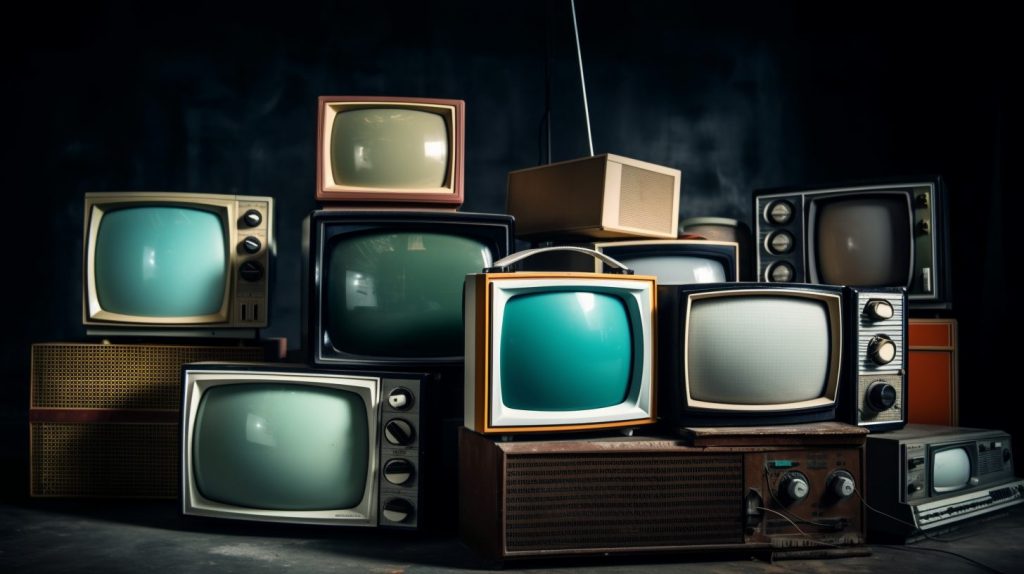
How Artificial Intelligence should understand videos
About Dr. Simone Schaub-Meyer
Dr. Simone Schaub-Meyer is an expert in computer vision and conducts research at the interface of computer vision, computer graphics and machine learning.
Schaub-Meyer completed her doctorate at ETH Zurich in 2018 in collaboration with Disney Research Zurich. The scientist then conducted research on augmented reality technologies as a postdoc at the Media Technology Lab at ETH Zurich.
In 2020, she moved to the Visual Inference Lab at TU Darmstadt as a postdoc. Since 2021, she has been the Junior Research Group Leader of the “data-Efficient Video Analysis” (EVA) group there.
EVA was founded as a DEPTH research group as part of the hessian.AI cluster project “The Third Wave of Artificial Intelligence – 3AI” funded by the hessian Ministry of Science and the Arts.
AI video analytics to be data and computationally efficient
Dr. Simone Schaub-Meyer is researching data-efficient, robust and controllable methods of video analysis, i.e. methods that extract data from videos and can then be used for video interpolation, for example.
Their methods should be efficient in two areas, explains Schaub-Meyer: they have high computational efficiency – because at a resolution of 4K, for example, huge amounts of data have to be processed – and they understand video content with as few annotations as possible.
These annotations are usually created manually by humans to make the image content understandable for computers. For example, in an image showing a cat on a table, both objects are labelled “cat” and “table”. This method enabled large datasets like ImageNet and thus the triumph of supervised machine learning in computer vision.
Modern AI methods independently search for patterns in huge data sets
In the meantime, however, self-supervising methods have become established in which AI models are trained with billions of images without manually created labels and then fine-tuned to their respective field of application using specialised data sets with labels.
Schaub-Meyer is researching how such algorithms are used in video analysis and how they can learn with fewer labels.
Without labels, other signals are needed for learning. Schaub-Meyer is therefore researching methods with which temporal relationships in videos can be efficiently and robustly extracted, represented and used for various applications, such as representing movements in video analysis, synthesising new video images or segmenting and tracking objects in videos.
She also wants to investigate such representations in the diffusion models that are currently in widespread use, such as stable diffusion. Such diffusion models have many advantages, but also problems, says Schaub-Meyer. The researchers now need to find out what such models understand, what biases exist in the network, what problems they can solve and where their limits lie.
AI must become more interpretable
Her EVA research group was founded by hessian.AI. Schaub-Meyer appreciates the interdisciplinary collaboration that the centre facilitates, the exchange with other researchers and the financial support.
The scientist sees a major challenge in developing models that “really solve the problem and don’t do something unpredictable”. To do this, she says, a better understanding of such large AI models must be developed, their interpretability improved and the models made more robust. In this way, trust in the models can also be strengthened – a central challenge if they are to be used in critical areas.