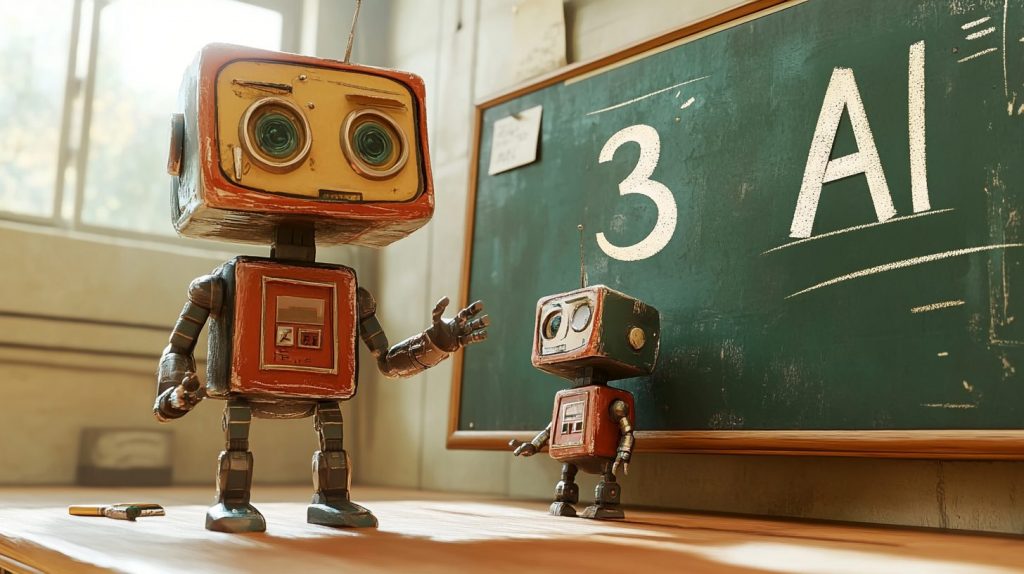
How object-centred learning makes AI agents better
About Jannis Blüml
Blüml already focussed on the field of reinforcement learning during his bachelor’s and master’s degree in computer science with an additional master’s degree in internet and web-based systems. His path eventually led him to the 3AI project of hessian.AI, a research cooperation focussing on AI. He is currently working on his doctorate under Prof Kristian Kersting, head of the Artificial Intelligence and Machine Learning Lab at TU Darmstadt and co-director of hessian.AI.
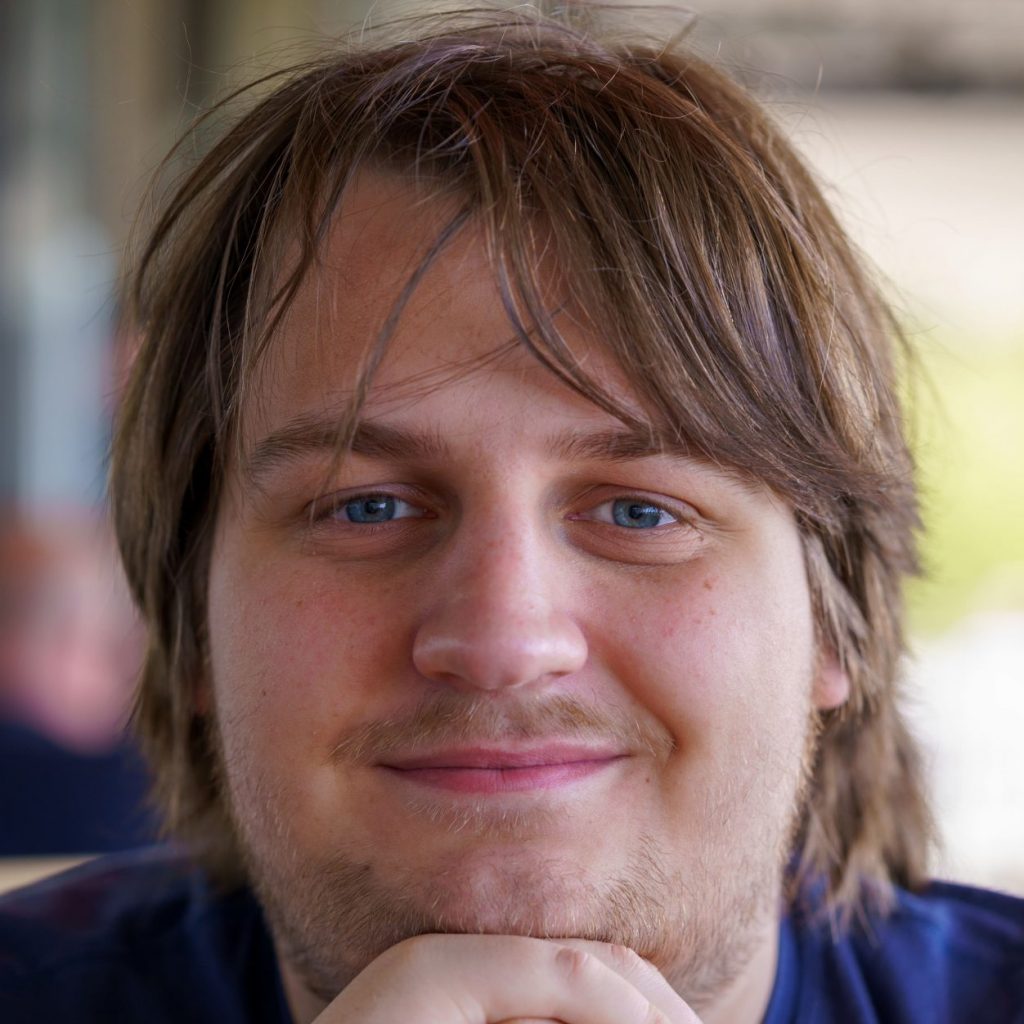
Jannis Blüml
3AI project and reinforcement learning agents
As part of the 3AI project, Blüml works closely with colleagues from various research groups. This interdisciplinary collaboration offers him different perspectives and approaches for his research. ‘There are of course many opportunities for cooperation, colleagues who look at the problem with different eyes and perhaps have different ideas for the solution.’
Blüml’s research is currently focused on improving the efficiency, generalisability and reasoning of reinforcement learning agents. In RL, agents act with their environment and learn through trial and error and through the feedback it receives from its environment. In his research, he deals with the question of how these environments can and should be represented.
Which environmental information is really important?
A central element of this is “object-centred learning”, in which important objects are brought to the fore and unimportant background information is suppressed. Blüml explains: ‘I simply ask myself here, how can I explain to the agent which information is important and which is not?’
Blüml illustrates this approach using the classic game of Pong as an example. Would it be sufficient to present the agent with just the bats and the ball instead of the entire playing field? The aim is to reduce the flood of information and concentrate on the relevant features in order to improve the reasoning and explainability of agents.
From the game board to the real world
Ultimately, his research is about developing algorithms that work well in different environments, even if not all information is available. Blüml relies primarily on classics such as the Atari 2600 to test his approaches, as it provides a wide range of different environments. His research aims to improve the robustness, explainability and generalizability of RL agents through an efficient and precise representation of the environments, e.g. using objects.
One challenge is always the applicability to the real world. Examples of how reinforcement learning research can influence the real world are Google DeepMind’s “AlphaFold” and “AlphaTensor”, which Blüml cites as prominent examples. Both show how insights from games such as Go and chess can be used to solve real-world problems, be it protein folding or matrix multiplication.
“That was another moment that confirmed for me that yes, games are also suitable for science. The insights we can gain from games can of course be transferred to the real world and to real problems.
According to Blüml, object-centered approaches can then bring benefits in a wide range of areas, from robotics to autonomous driving.