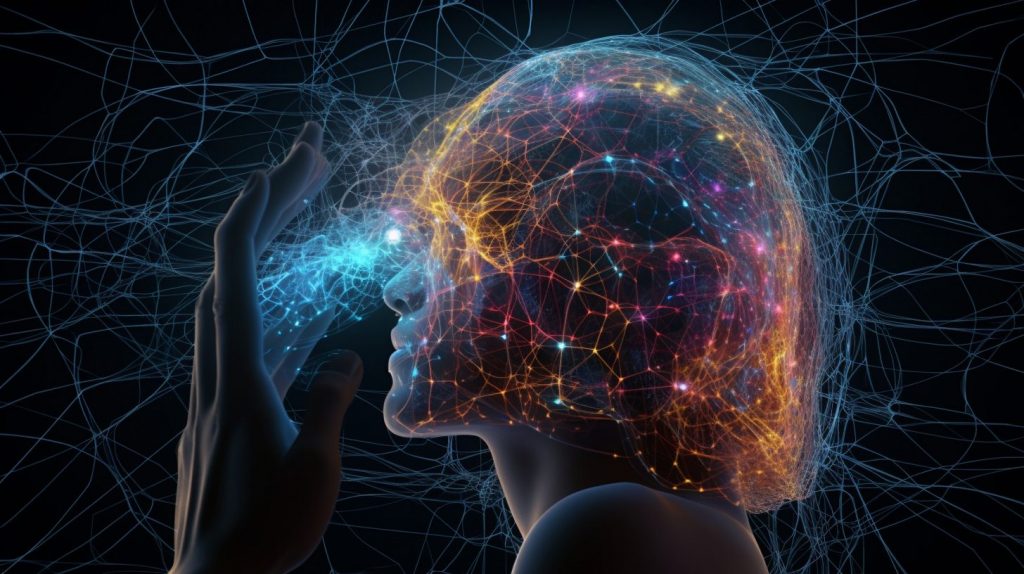
Why safe AI needs a holistic systems approach
About Prof. Dr. Visvanathan Ramesh
Prof. Dr. Visvanathan Ramesh is Professor of Software Engineering with a focus on “Bio-inspired Vision Systems” at the Goethe University Frankfurt and heads the “Center for Cognition and Computation” there.
After studying in India and the USA, he completed his doctorate in 1995 at the University of Washington on systematic methods for quantifying the limits of image processing systems. Ramesh then moved from academia to industry: from 1995 to 2011 he worked at Siemens Corporate Research in Princeton, where he rose from technical staff to Global Technology Field Leader. His focus was on real-time vision systems and machine vision.
In 2011, Ramesh followed the call to Frankfurt. His current research focus is transdisciplinary research in the field of systems science and the development of intelligence.
The Systems Approach to Artificial Intelligence
Over the past decade, the increasing availability of Big Data and AI tools has significantly accelerated the development and application of AI systems in the real world – and with it the need for methods to make such AI systems safe.
For Prof. Dr. Visvanathan Ramesh, the solution to this task lies in a holistic, transdisciplinary systems perspective that combines traditional model-based thinking with modern, data-driven machine learning.
In doing so, Ramesh builds on a thirty-year research base and develops scalable AI designs that map the context of the respective “world”, the tasks and the performance requirements to transparent, explainable and cognitive architectures for each application domain.
For example, Ramesh is researching, among other things, how AI systems can be developed for real-world use in various industries. Statistical methods for image processing from Deep Learning are an important part of this – but more is needed.
“My research has always been about how to develop machine vision systems in a principled way, with a clear understanding of where the limitations of the system are,” says Ramesh. “Ultimately, the system is built for a specific purpose.”
This requires a holistic systems approach that models the world in which the system is intended to function – including the questions the system is intended to answer and the performance it is intended to deliver.
“Such systems also need to communicate when they stop working,” says the scientist. Only in this way are robust AI systems for real-world use possible.
Classical engineering methods & neural networks
In his research, Ramesh models application domains – or worlds – for different problems. One example: the automated inspection of a bridge by a drone looking for cracks in the structure.
“I have certain principles according to which I can design the system. In the bridge example, that means something like: what are their properties, what will I see there, what camera sensors do I use and so on,” says Ramesh. “I can pull this information from science, for example, and use it to construct a clear picture of a contextual model of this ‘world’.”
The model can then be used to infer, for example, which images the AI system will see, which are important and which can be ignored.
In doing so, the variables that are relevant to the problem are just as important as those that are not – so the system knows what to look out for. He and his team then build causal and probabilistic models. Modern deep-learning methods such as unsupervised learning can help with the latter, for example in simulations.
“We combine classical engineering methods with neural networks,” the scientist explains. The resulting systems are often also called hybrid AI systems.
Ramesh also exchanges ideas on this topic with other scientists at hessian.AI, such as Prof. Dr Kristian Kersting and Prof. Dr Constantin Rothkopf, both from TU Darmstadt.
Continuous learning systems and human-machine interactions
Ramesh describes the development of intelligent architectures with an algorithmic core that enable continuous learning systems as a central challenge. Continuous learning is a topic that other hessian.AI researchers are also working on, including Martin Mundt, who heads the “Open World Lifelong Learning” group at the TU Darmstadt and hessian.AI and has a PhD from Ramesh’s “Center for Cognition and Computation”.
He also sees the integration of humans and machines as an important task in the long term: “AI and humans should resonate with each other, we should integrate naturally, complement each other and develop together,” says the scientist. AI must understand humans and humans must understand AI.
His work is to embed AI systems in context so that they do their job robustly and predictably. This way, they can be used safely in critical areas such as healthcare, and in the future, even the increasingly scaling systems can still remain verifiable, Ramesh concludes.