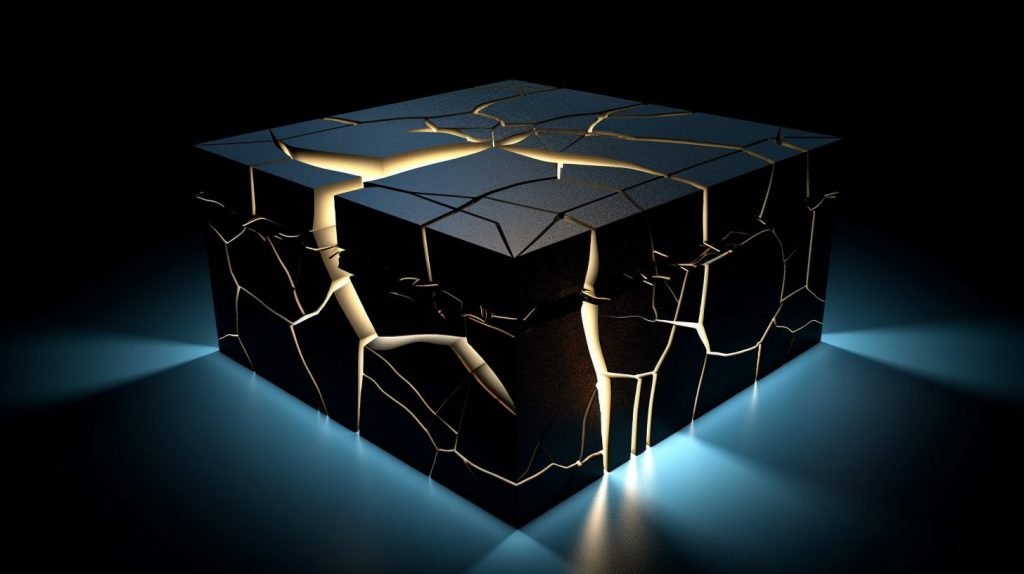
Cracking the Black Box: Understanding and Preventing Catastrophic Forgetting in AI
About Roshni Kamath
Roshni Kamath is a PhD student at Technische Universität Darmstadt. She completed her bachelor’s degree in information technology software engineering at the University of Mumbai and holds a master’s in Artificial Intelligence at Katholieke Universiteit (KU) Leuven.
Prior to joining the Open World Lifelong Learning research group at TU Darmstadt in June 2022, she worked as a software engineer, and was an AI researcher at Forschungszentrum Jülich.
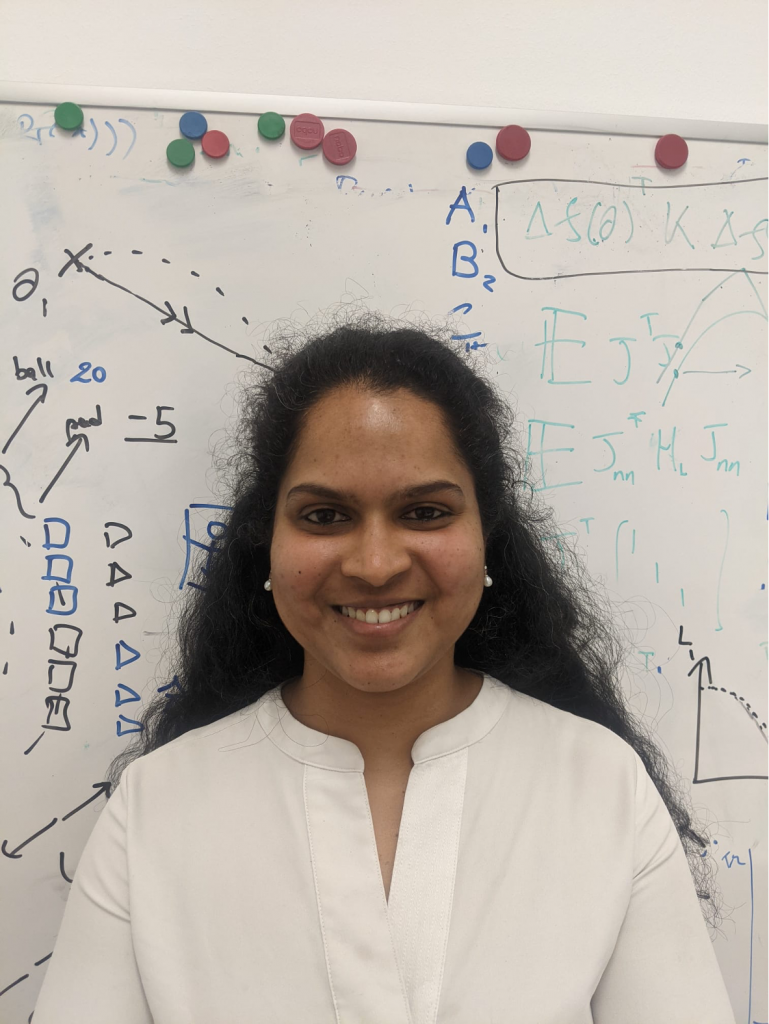
Emulating human learning in AI
Imagine you are learning to play a musical instrument. As you progress, you learn new songs and techniques. At the same time, you’re likely to remember and be able to play the pieces you’ve learned before.
This ability to accumulate and retain knowledge and skills over time is a fundamental aspect of human learning. To bring this same capability to AI systems, Kamath’s research focuses on “continuous learning in AI.”
Her goal is to better understand the mechanisms of AI models and optimize them to efficiently incorporate new information into existing knowledge, thereby improving their performance over time.
One aspect of her research addresses a major challenge in continuous learning: “catastrophic forgetting.” When a model trained on one task is presented with new data for a different task, it can forget what it has learned. This leads to a drop in performance. Kamath’s research focuses on understanding this phenomenon and finding ways to prevent it.
For example, when training a model with brain images from different hospitals, variations in resolution, metadata, and acquisition process can affect model performance and catastrophic forgetting. Kamath wants to go beyond simply measuring the accuracy of an AI model and focus on the multifaceted factors that can influence learning and forgetting, an approach that aims to resemble real life.
“The objective of my work is to create new techniques and establish links between different machine learning paradigms,” says Kamath. Her goal is to produce advanced AI systems that possess the ability to independently learn in an unbounded environment. These systems can learn continually, detect novel situations successfully, and decide on the data to use for training.
In this context, Kamath’s research involves teaching models about themselves, the data on which they were trained, and what they are capable of. This is part of creating a framework for models to prevent catastrophic forgetting in open-world lifelong learning scenarios.
Advancing continuous learning in AI is a balancing act
For Kamath, this means balancing different approaches and taking a holistic view. Transfer learning, for example, enables AI models to leverage knowledge from previous problems as they face new challenges. By transferring knowledge between tasks, AI systems can adapt more efficiently to new data and situations without having to start from scratch.
According to Kamath, this can also raise concerns, for example in data privacy. She notes: “In a hospital setting, many patients are not comfortable with storing or sharing their data. Fine-tuning a model for a new task by transferring knowledge can risk inadvertently leaking information from previous tasks, even if it was anonymized or initially non-sensitive.
Her research aims to find a balance, particularly given the impact of lifelong learning in AI systems on energy consumption. Improving the performance of models can lead to energy savings and more sustainable AI systems, particularly those involving large-scale machine learning models that require significant computational resources.
For example, transfer learning can reduce the amount of training time and computational resources required for each new task by using pre-trained models or shared knowledge between tasks, which can save energy. Kamath regularly attends meetups with the hessian.AI network, sharing ideas with other researchers and learning about their challenges. These interactions provide valuable insights into the AI community’s questions and allow her to improve and focus her research, furthering the development of continuous learning in AI.