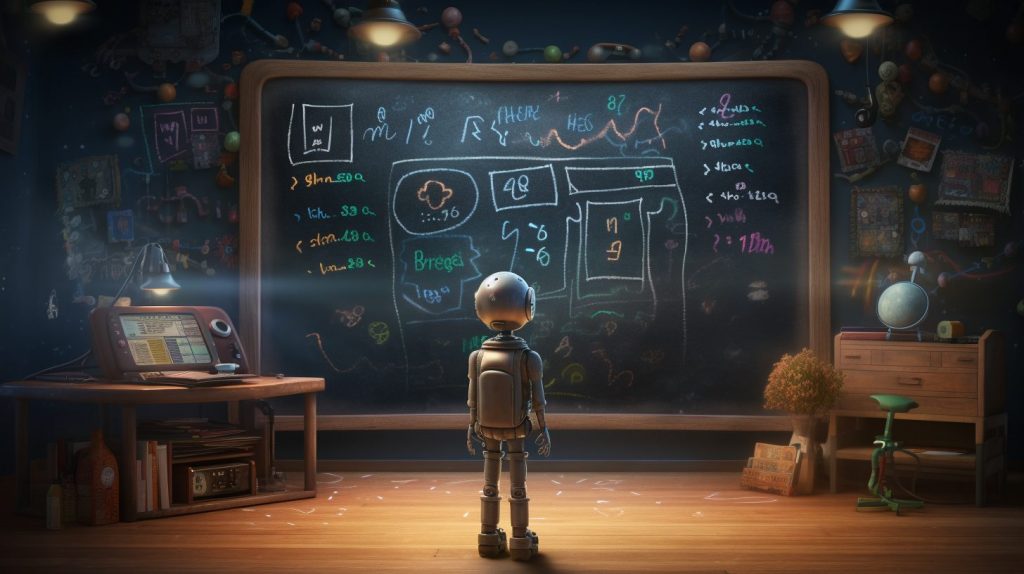
Equation Discovery: How AI could automate scientific discovery
About Jannis Brugger
Jannis Brugger studied natural sciences and computer science in Koblenz and Mainz, where he began to work on artificial intelligence.
He is currently doing his PhD at hessian.AI as part of the 3AI (The Third Wave of AI) project.
Automated scientific discovery
Jannis Brugger’s speciality is equation discovery, which involves deriving mathematical formulae from data sets.
Brugger gives a simple example: “If we have a data set with two bodies of mass, for example, we can try to derive the laws of gravity from it.”
Other fields of application are materials science, where better batteries could be developed, for example, or biochemistry, where formulas could be found that describe how molecules assemble.
Equation Discovery could therefore become a standard tool for scientists in the future, analysing data from thousands of experiments and generating a set of formulae that scientists can then study.
Grammar sets the rules for AI
What is special about Brugger’s research is the neuro-symbolic focus: in his work, he combines neural networks with rules that describe the formation of formulae, i.e. a grammar.
The neural network analyses the data and searches within the grammar for new formulas that fit well with the patterns in the data.
One advantage of this method is that the researcher can interpret and transform the formulas found – and the network only outputs well-formed formulas.
This distinguishes his approach from other methods that, for example, rely exclusively on transformer models such as those on which ChatGPT is based.
The use of such models is promising, but the lack of a grammar cannot, for example, prevent the AI model from outputting incorrect syntax, such as generating an addition sign several times in a row. The grammar, on the other hand, provides a clear search space that the model explores.
In addition, the grammar theoretically allows the integration of domain knowledge – that is, the knowledge that scientists have already gathered about a research area. This narrows the search space and can thus speed up the discovery of new, useful formulas.
Real world data remains a challenge
He says his position at hessian.AI allows him to interact with various experts from other fields in his work; the 3AI project now has more than a dozen PhD students working in different research groups.
The different groups often have a unique perspective on similar problems, he says, for example, there is overlap between equation discovery, programme synthesis and proof finding.
Brugger sees a major challenge in his work in dealing with “noisy data”, i.e. data from the real world that contain measurement inaccuracies or come from different sources.
Successful Equation Discovery is therefore still limited to “perfect data” and further research is needed to realise the promise of the method.
If successful, Equation Discovery could change science – and thus our society – forever.