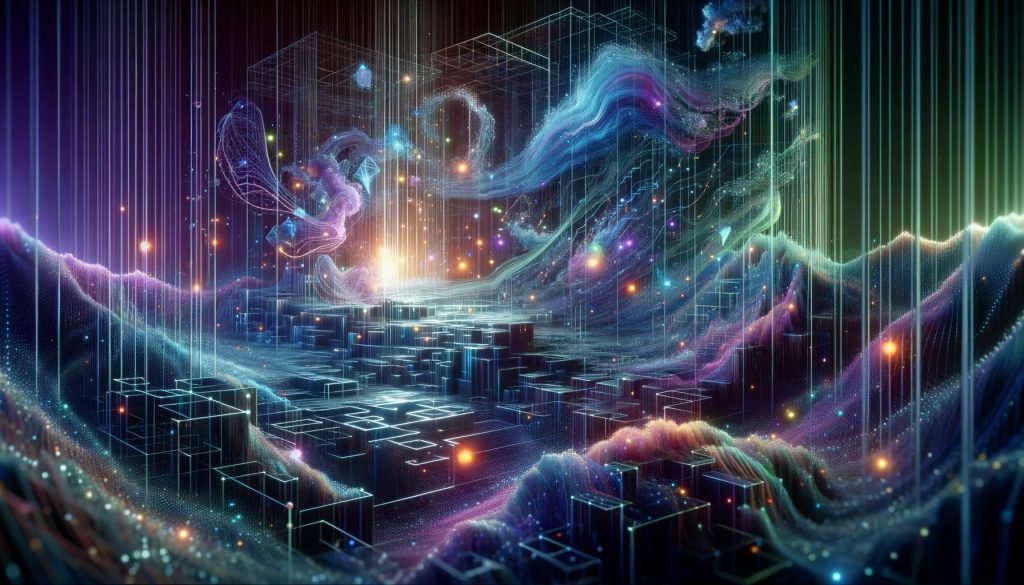
How Synthetic Data and Simulations Advance Autonomous Tech
About Prof. Dr. Dominik L. Michels
After studying computer science and physics and completing his doctorate at the University of Bonn, Dominik L. Michels joined Caltech as a postdoc. In autumn 2014, he became head of the High Fidelity Algorithmics Group at the Max Planck Center for Visual Computing and Communication at Stanford University.
Today, Michels holds the chair (W3) for Intelligent Algorithms in Modeling and Simulation (IAMS) at the Technical University of Darmstadt and is an Associate Professor at KAUST, where he established the Computational Sciences Group in the summer of 2016.
Mathematical modeling, synthetic data, and simulation
Michels’ research revolves around the central topic of mathematical modeling and numerical simulation, with the aim of digitally mapping systems and investigating underlying phenomena. This interface between mathematics, physics, and computer science allows for translating complex processes in the real world into digital models. Michels explains, “It’s actually always about modelling a system digitally. You could also call this a digital twin”.
One focus of Michels’ work is the creation and utilisation of synthetic data for training AI systems. Such data can be helpful in cases where very few real data is available, such as edge cases in autonomous driving. Synthetic data offers a cost-effective and flexible alternative, as Michels emphasizes: “Computer-aided simulations generate synthetic data that is automatically labelled or annotated.”
As an example, he cites automated processes in agriculture, where entire vegetation growth processes have to be simulated, as this application requires not just a few, but thousands of different data points.
Michels’ research also focusses on the question of how AI can improve numerical simulations. Many simulations are computationally intensive and iterative – the algorithms are faster the closer the starting point of the simulation is to the desired end state. Here, AI can help by bringing the starting point of the iterative process closer to the end state. Such faster and better AI-supported simulations would have applications in material sciences, chemistry, and physics – and thus in many industries.
Hessian.AI unique in Germany
Michels also sees this intertwining of research and entrepreneurial activities at Hessian.AI. The center is unique in this configuration in Germany, according to Michels. Hessian.AI also places a special focus on technology transfer. He tells me about one of his lectures on technology transfer and entrepreneurship with a focus on AI.
“You can tell that many of the students who now have to write a business plan and make a pitch deck as their final assignment in my lecture have more or less already learnt these things in the Hessian.AI ecosystem or have at least come into contact with them.”
“Lack of Data” holds back 80 percent of all AI projects
In practice, Michels sees the biggest challenge in the “Lack of Data”. Many AI projects fail or do not reach their full potential because suitable, structured, and annotated data are lacking – in well over 80 percent of all machine learning projects, data shortage is a problem. Here, Michels sees a crucial starting point for research: “It is important not only to address the network structures, but also to ask where the data comes from.”
The advances of the last two years in the field of generative AI, such as diffusion models, are very interesting here. The scientist sees great potential in the use of generative AI for enriching existing data. The systems could not yet generate entirely new data.
Great Potential for the “Old Economy”
Synthetic data could also play a key role in regions with stricter data protection laws such as Europe, explains Michels.
Especially in Germany and Central Europe, there are fewer digital services and more traditional, physically product-oriented business – the “Old Economy.” Overcoming the “Data Bottleneck” could unlock much potential here by automating many processes.
“This would also free up resources in the affected industries to think about new, modern business models,” says Michels.